Data annotation tech reviews
Data annotation technology plays a pivotal role in the realm of machine learning and artificial intelligence. It involves the process of labeling raw data to make it understandable for machines, thereby facilitating the training of algorithms. This article explores the significance of data annotation, its various techniques, applications across different industries, challenges faced, best practices, future trends, and case studies showcasing its effectiveness.
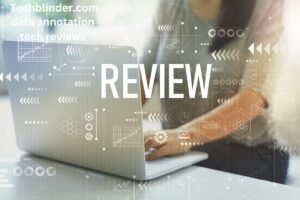
Introduction to Data Annotation Technology
Definition and Importance
Data annotation refers to the process of labeling data with relevant metadata or tags to make it understandable for machines. It is a crucial step in the development of machine learning models as it provides the necessary context for algorithms to learn patterns and make accurate predictions.
Evolution of Data Annotation Technology
Over the years, data annotation technology has evolved significantly, driven by advancements in machine learning algorithms, computing power, and the availability of large datasets. From manual annotation methods to sophisticated automated tools, the landscape of data annotation has transformed, enabling more efficient and accurate data labeling.
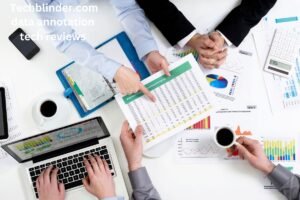
Types of Data Annotation Techniques
Manual Annotation
Manual annotation involves human annotators labeling data manually based on predefined guidelines. Although labor-intensive and time-consuming, manual annotation ensures high accuracy, especially for complex tasks that require human judgment.
Semi-automatic Annotation
Semi-automatic annotation combines human expertise with machine assistance. It involves using annotation tools that automate certain aspects of the labeling process while allowing human annotators to intervene when necessary, thereby improving efficiency without compromising accuracy.
Automatic Annotation
Automatic annotation utilizes machine learning algorithms to label data automatically without human intervention. While it offers unparalleled speed and scalability, automatic annotation may lack the precision and context provided by human annotators, especially for nuanced tasks.
Applications of Data Annotation
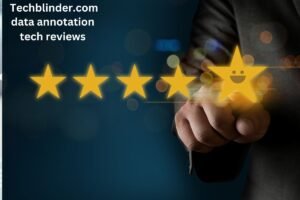
Data annotation technology finds applications across various industries, including:
Machine Learning and AI
In machine learning and AI, annotated data is essential for training algorithms to recognize patterns and make predictions. Applications range from image recognition and natural language processing to sentiment analysis and recommendation systems.
Autonomous Vehicles
Data annotation plays a critical role in the development of autonomous vehicles by labeling sensor data such as images, LiDAR scans, and radar signals to enable real-time decision-making and navigation.
Natural Language Processing
In natural language processing, annotated datasets are used to train models for tasks such as named entity recognition, sentiment analysis, and language translation, enabling machines to understand and generate human-like text.
Healthcare
In healthcare, data annotation technology is used to label medical images, patient records, and genomic data, facilitating tasks such as disease diagnosis, treatment planning, and drug discovery.
E-commerce
In e-commerce, annotated data helps improve product recommendations, personalize user experiences, and optimize search results, leading to increased sales and customer satisfaction.
Challenges in Data Annotation
Despite its importance, data annotation poses several challenges, including:
Quality Control
Ensuring the accuracy and consistency of annotations can be challenging, especially when dealing with large datasets and complex labeling tasks.
Scalability
Scaling up data annotation processes to handle large volumes of data requires efficient workflows, robust infrastructure, and skilled annotators.
Cost-effectiveness
Data annotation can be costly, particularly for tasks that require domain expertise or specialized tools. Balancing cost and quality is essential to ensure the affordability of annotation projects.
Security and Privacy Concerns
Annotating sensitive or confidential data raises concerns about data privacy and security. Implementing robust security measures and compliance with regulations are imperative to safeguarding sensitive information.
Best Practices in Data Annotation
To overcome these challenges and maximize the effectiveness of data annotation, organizations should follow best practices such as:
Choosing the Right Annotation Tool
Selecting annotation tools that are tailored to specific tasks and datasets can improve efficiency and accuracy.
Establishing Annotation Guidelines
Defining clear annotation guidelines and providing comprehensive training to annotators ensure consistency and quality in labeling.
Continuous Training and Evaluation
Regular training sessions and performance evaluations help maintain annotators’ proficiency and identify areas for improvement.
Collaborative Annotation
Encouraging collaboration among annotators and domain experts fosters knowledge sharing and ensures accurate annotations across diverse datasets.
Future Trends in Data Annotation
Looking ahead, several trends are shaping the future of data annotation, including:
Advancements in AI for Annotation
The development of AI-powered annotation tools capable of understanding and labeling data autonomously is expected to streamline the annotation process and improve efficiency.
Integration of Machine Learning Algorithms
Integrating machine learning algorithms directly into annotation tools can enhance their capabilities, enabling adaptive labeling and automatic quality control.
Crowdsourcing and Distributed Annotation
Crowdsourcing platforms and distributed annotation frameworks allow organizations to tap into a global pool of annotators, enabling faster turnaround times and scalability.
Case Studies
Successful Implementations
Case studies highlighting successful implementations of data annotation technology across various industries demonstrate its impact on enhancing machine learning models’ performance and driving innovation.
Lessons Learned
Examining lessons learned from past annotation projects helps organizations avoid common pitfalls and refine their annotation strategies for future endeavors.
Conclusion
Data annotation technology plays a vital role in the development and deployment of machine learning models across diverse industries. By providing contextual information to algorithms, data annotation enables machines to learn from data and make informed decisions. However, overcoming challenges such as quality control, scalability, and cost-effectiveness requires adopting best practices and embracing emerging trends in annotation. As technology continues to evolve, data annotation will remain a cornerstone of AI-driven innovation, driving advancements in fields ranging from healthcare and autonomous vehicles to e-commerce and natural language processing.
Unique FAQs
- What are the key considerations when choosing a data annotation tool?
- Factors such as task complexity, dataset size, annotation type, and budget play crucial roles in selecting the right annotation tool for a project.
- How can organizations ensure the quality of annotated data?
- Implementing robust quality control measures, providing comprehensive training to annotators, and conducting regular evaluations are essential for ensuring the accuracy and consistency of annotated data.
- What role does data privacy play in data annotation projects?
- Data privacy is a significant concern in data annotation projects, especially when dealing with sensitive or confidential information. Organizations must implement stringent security measures and comply with relevant regulations to protect data privacy.
- How can organizations address the scalability challenge in data annotation?
- Leveraging automation technologies, crowdsourcing platforms, and distributed annotation frameworks can help organizations scale up their annotation processes to handle large volumes of data efficiently.
- What are the emerging trends in data annotation technology?
- Emerging trends in data annotation technology include advancements in AI-powered annotation tools, integration of machine learning algorithms for adaptive labeling, and the use of crowdsourcing and distributed annotation for scalability.
- Emerging trends in data annotation technology include advancements in AI-powered annotation tools, integration of machine learning algorithms for adaptive labeling, and the use of crowdsourcing and distributed annotation for scalability.
1 thought on “data annotation tech reviews”